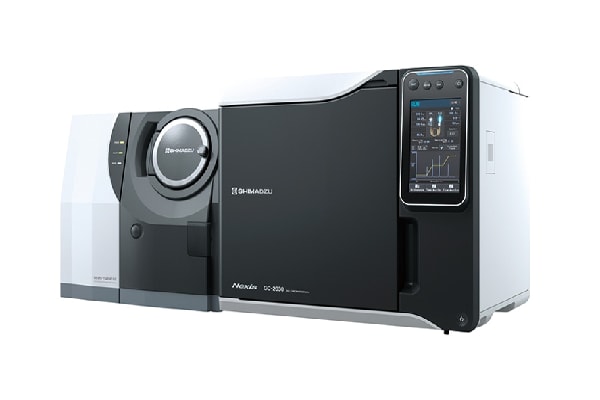
GCMS-TQ™ 8040 NX
- The Smart Aroma Database helps detect low-concentration aroma components in MRM mode. - The Multi-Omics Analysis Package can detect particle size concentrations and sizes contributing to differences between samples, using both primary metabolite and particle measurement data.
In recent years, the demand for vegetable juices has increased with the rise of health awareness. As more people demand a health-conscious diet, vegetable juices are popular because they are nutrient-rich and easy to consume. However, there are a variety of vegetable juices on the market, and consumer tastes are diversifying. For example, there are various kinds of vegetable juices, such as vegetables that combine tomatoes, cabbage, and carrots, and smoothies that combine fruits and vegetables. Therefore, in developing vegetable juices, it is necessary to provide products tailored to consumers' tastes and needs. Analyzing particle size and aroma concentration is crucial in developing vegetable juices. Large grain size can affect palatability and ease of drinking, while inappropriate aroma concentration can lead to an unattractive flavor. Quality and taste of vegetables may vary due to seasonal changes, cultivation methods, soil and climate conditions. This presents a challenge for product developers who require an objective evaluation method. This application presents an example of measuring 4 types of commercially available vegetable juices using the dynamic particle image analysis system iSpect DIA-10 and the gas chromatograph mass spectrometer GCMS-TQTM 8040 NX equipped with AOC-6000Plus. Particle size distribution was measured using a 300 μm cell (special type) to evaluate particle concentration and shape. GC/MS measured 484 aroma components using the Smart Aroma Database. The results were integrated and analyzed using the Multi-Omics Analysis Package (Garuda).
February 27, 2024 GMT
Some products may be updated to newer models